PROST: Physical Reasoning of Objects through Space and Time
A probing dataset highlighting why the lack of real-world experience hinders language understanding.
In the context of natural language processing (NLP), Bender and Koller (2020) provide a working definition of “understanding” as the ability to recover the communicative intent from an utterance. To achieve this, one must be able to query a set of concepts that is aligned with the speaker’s own understanding. An example of such alignment is interaction with the physical world, an experience shared by all humans and that provides a common set of concepts to rely on in communication. For example, the reader can map the phrase “I dropped my pint glass}]”” to a set of relevant experiences and generate a mental depiction of the scene. Further, the reader can also use their knowledge of gravity and the properties of a pint glass to reason about potential outcomes: the pint glass will fall toward the ground and will likely break on impact.
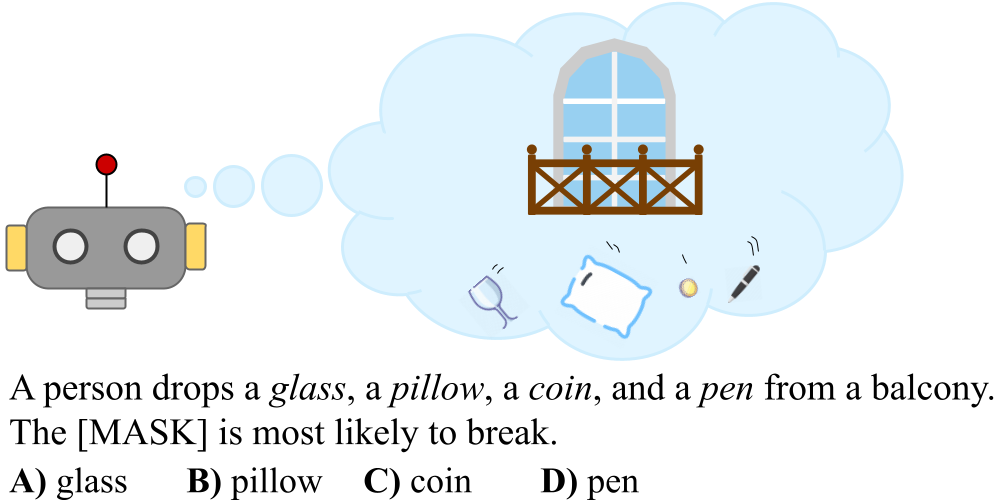
Children grab, push, and play with the objects around them to form concepts about the world they live in even before learning to talk. These concepts are linked with words to enable communication, eventually providing the necessary grounds for concepts and language to co-develop. In contrast, current language models (LMs) are not exposed to real-world experiences, making them incapable of grounding language. We hypothesize that this lack of experience impedes their ability to both understand an utterance relating to the physical world, and their ability to reason about its implications. For this reason, we develop PROST: Physical Reasoning about Objects Through Space and Time, a dataset created to probe a language models physical reasoning. It contains 18,736 multiple-choice questions made from 14 manually curated templates, covering 10 physical reasoning concepts. All questions are designed to probe both causal and masked LMs in a zero-shot setting.
We conduct an extensive analysis of modern language models on PROST, which demonstrates that state-of-the-art pretrained models are weak at physical reasoning: they are influenced by the order in which answer options are presented to them; they struggle when the superlative in a question is inverted (e.g. most <-> least); and increasing the amount of pretraining data and parameters only yields minimal improvements. These results provide support for the hypothesis that current pretrained models’ ability to reason about physical interactions is inherently limited by a lack of real world experience. By highlighting these limitations, we hope to motivate the development of models with a human-like understanding of the physical world.